Unlocking the Power of Image Datasets for Classification
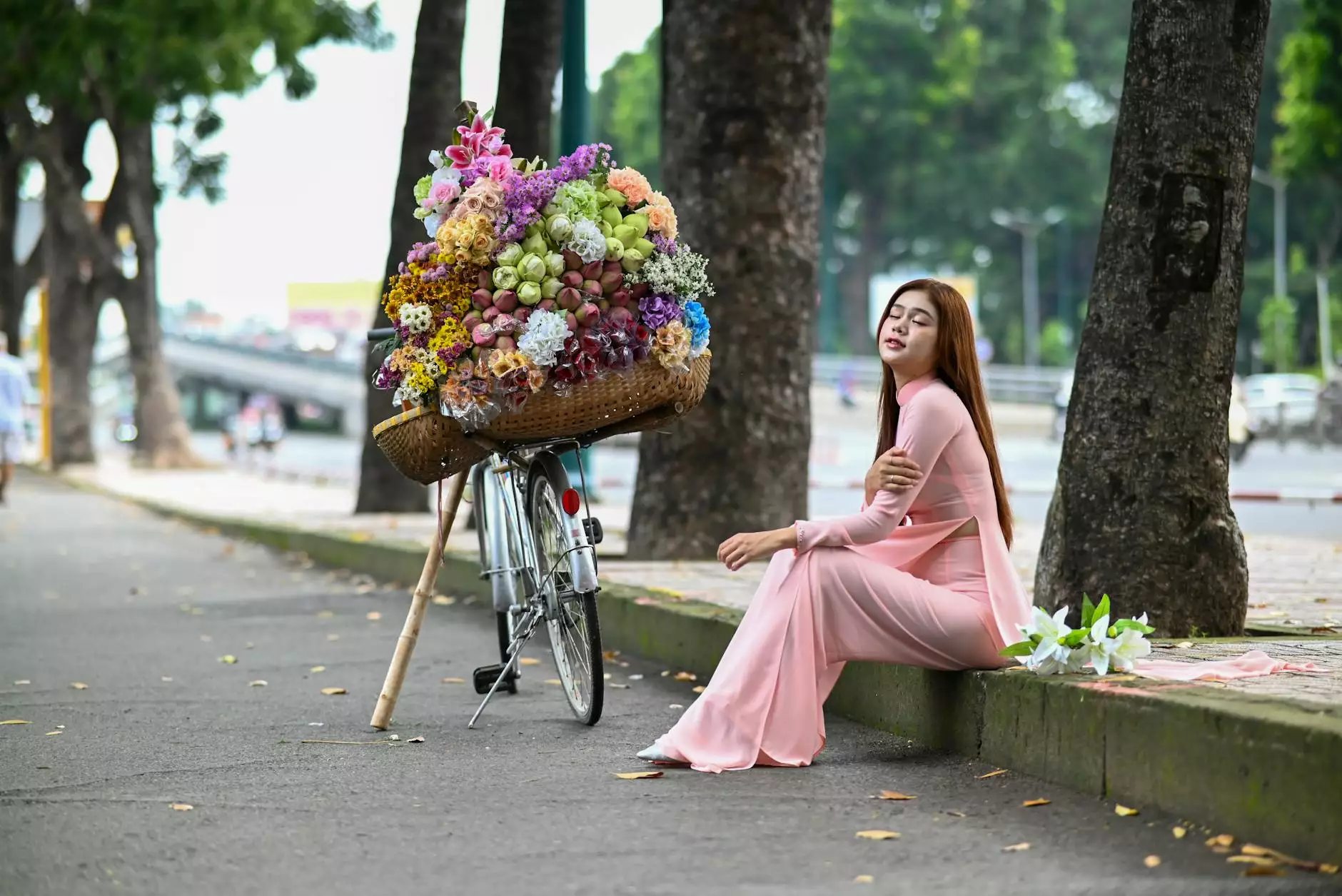
In today’s data-driven world, image datasets for classification are playing a pivotal role in various sectors, including healthcare, automotive, and finance, among others. They not only facilitate the development of innovative solutions but also empower businesses to make informed decisions through advanced machine learning and artificial intelligence techniques. This article delves into the significance of image datasets for classification, the role of data annotation tools, and how platforms like KeyLabs.ai are transforming the landscape.
Understanding Image Classification
Image classification is a crucial process in which algorithms are trained to recognize and categorize images into predefined labels. This fundamental aspect of computer vision is essential for various applications, including facial recognition, object detection, and automated quality control in manufacturing.
The Importance of High-Quality Datasets
At the heart of effective image classification lies the quality of the dataset used. High-quality image datasets enable machine learning models to achieve greater accuracy and efficiency. Key attributes of a robust dataset include:
- Diversity: A wide range of examples across different categories improves the model's generalization capabilities.
- Quantity: Large volumes of data help in training more sophisticated models that can recognize subtle patterns.
- Label Accuracy: Precise and consistent labeling is crucial for training algorithms to differentiate between classes effectively.
Key Components of Image Datasets for Classification
When constructing image datasets for classification, several components must be considered to enhance the dataset's effectiveness:
1. Data Collection
Gathering images from relevant sources is the first step in building a comprehensive dataset. Sources of images can include:
- Public datasets such as ImageNet and CIFAR-10.
- Web scraping from various online platforms.
- In-house images collected from company operations or product photography.
2. Data Annotation
Data annotation is the process of labeling images, which could involve categorizing images, drawing bounding boxes around objects, or segmenting different regions within an image. This step is vital because incorrect or inconsistent annotations can lead to poor model performance.
3. Preprocessing Data
Before using the dataset for training models, it is essential to preprocess the images. This can involve:
- Resizing images to a consistent size.
- Applying data augmentation techniques like rotation, flipping, or adding noise to increase variability.
- Normalizing pixel values to improve model convergence during training.
4. Splitting the Dataset
To evaluate the performance of image classification models accurately, the dataset should be divided into training, validation, and test sets.
The Role of Data Annotation Tools
Data annotation tools are essential in creating high-quality image datasets for classification. They simplify the annotation process, allowing for efficiency and accuracy. KeyLabs.ai provides advanced tools that streamline this process, offering features such as:
- User-Friendly Interface: Intuitive design that allows both technical and non-technical users to annotate images effortlessly.
- Collaboration Features: Tools for teams to work together in real-time, ensuring a more consistent and faster annotation process.
- Customizable Workflows: Capabilities to tailor the workflow according to specific project needs.
Choosing the Right Data Annotation Platform
Selecting the right data annotation platform can significantly impact the quality of the datasets created. KeyLabs.ai stands out due to its innovative features designed specifically for dataset enrichment:
- Scalability: Ability to handle large datasets efficiently, enabling businesses to grow without compromising on quality.
- Integration Capabilities: Seamless incorporation of the annotation platform with existing workflows and machine learning frameworks.
- Quality Assurance Mechanisms: Built-in checks to ensure that the annotations meet the required standards for optimal model training.
Case Studies: Successful Implementation of Image Datasets for Classification
Various companies have leveraged image datasets for classification to enhance their services and improve operational efficiency. Here are a couple of case studies showcasing this trend:
1. Healthcare Industry
In the healthcare sector, companies have employed image classification algorithms to analyze medical images, such as X-rays and MRIs. By using annotated datasets, machine learning models have been able to identify anomalies, improving diagnostic accuracy and treatment planning. Companies like Siemens Healthineers and Zebra Medical Vision have successfully implemented such solutions, significantly reducing the workload on medical professionals.
2. Retail Sector
Retailers are utilizing image classification for various applications, including inventory management and customer behavior analysis. By analyzing product images and customer interactions, businesses can optimize stock levels and personalize marketing strategies. Notable platforms like Amazon and Walmart use advanced image recognition technology to enhance customer experiences and operational efficiency.
Future Trends in Image Classification and Datasets
As technology evolves, the landscape of image classification is also changing. Here are some trends to watch for in the coming years:
1. Deep Learning Advances
Deep learning continues to be at the forefront of image classification, with newer architectures evolving to provide better performance and accuracy. Techniques like transfer learning are becoming popular, allowing the reuse of existing models on new datasets.
2. Automation in Data Annotation
With the advent of artificial intelligence, automated data annotation is emerging as a viable option. While human oversight remains critical, AI-assisted tools can significantly speed up the annotation process, making it more feasible to manage vast datasets.
3. Ethical Considerations
As the use of image datasets grows, so does the importance of ethical standards in data collection and annotation. Ensuring privacy and preventing bias in datasets are critical to the responsible deployment of image classification technologies.
Conclusion
In summary, image datasets for classification are foundational to the development of effective machine learning models across various industries. Companies like KeyLabs.ai are instrumental in providing the necessary tools to create high-quality datasets through efficient data annotation processes. By recognizing the importance of high-quality datasets and leveraging appropriate annotation tools, businesses can enhance their competitive edge in the evolving landscape of artificial intelligence and machine learning. Embracing these advancements will not only lead to improved operational outcomes but also spur innovation across sectors.